Lunch Rush Hour: I read all the major LLM whitepapers so you don't have to
Pardon the sensationalist title, I'm just testing Substack's recommendation algorithm
I like the dances of meaning words do with one another, the endless changes and complexities of their interrelationships in sentence or text, by which imaginary worlds are built and shared. — Ursula Le Guin, “Having My Cake”
Attention Is All You Need (Google, 2017)
[N.B. Aidan Gomez cofounded Cohere and Illia Polosukhin pulled a total power move by using his @gmail.com
here.]
Neural networks are named as such because they are inspired by the neurons of the human brain. Neural networks represent neurons as nodes and interactions between neurons as mathematical and probabilistic computations. Just as in the human brain, networks of interlinked computational nodes are used to predict and reason.
For example, recurrent neural networks (RNNs) are deep (many-layered) neural nets with recursive properties (e.g. bidirectional, hidden layer computation to provide a form of short-term memory to the network) that are tuned to the general sequence to sequence problem [N.B. I see Sutskever, I click].
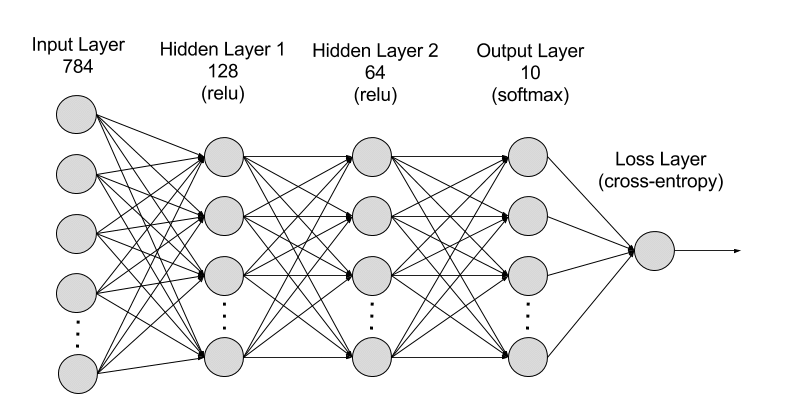
RNNs can therefore be well-suited for things like language translation and sequence generation [N.B. Chris Olah and Shan Carter described attention in the context of augmented RNNs (Neural Turing Machines with external vector array memory, content- and location-based attention) in 2016 at Google Brain: “Our guess is that these ‘augmented RNNs’ will have an important role to play in extending deep learning’s capabilities over the coming years.” They were right.].
In this work we propose the Transformer, a model architecture eschewing recurrence and instead relying entirely on [self-attention] to draw global dependencies between input and output. The Transformer allows for significantly more parallelization and can reach a new state of the art in translation quality after being trained for as little as twelve hours on eight P100 GPUs.
Self-attention “allows the model to identify and weigh the importance of different parts of the input sequence by attending to itself” e.g. linearly transforming input sequences to queries, keys (in the paper, both with dimension d_k) and values (dimension v_k), computing a weighted sum:
The authors justify the self-attention methodology (vs. RNN or ConvNet) defining the Transformer model architecture with a computational complexity argument:
…a self-attention layer connects all positions with a constant number of sequentially executed operations, whereas a recurrent layer requires O(n) sequential operations. In terms of computational complexity, self-attention layers are faster than recurrent layers when the sequence length n is smaller than the representation dimensionality d, which is most often the case with sentence representations used by state-of-the-art models in machine translations
Language Models are Few-Shot Learners (OpenAI, 2020); GPT-3
[N.B. This has one of the longer author lists I’ve seen and is a bit of an internet museum piece in 2024 with both Dario Amodei’s and Ilya Sutskever’s names featured prominently. OpenAI must have learned their lesson after this as they removed individual author attribution and requested the GPT-4 paper be cited as (OpenAI, 2023).]
This paper identified a task-specific data and fine-tuning limitation becoming more prominent especially as model architectures including fine-tuned transformer-based language models were able to become increasingly task-agnostic.
We use the same model and architecture as GPT-2, including the modified initialization, pre-normalization, and reversible tokenization described therein, with the exception that we use alternating dense and locally banded sparse attention patterns in the layers of the transformer, similar to the Sparse Transformer.
The authors propose a few potential evaluation settings for GPT-3 scaled generally to “how much task-specific data they tend to rely on”:
Fine-tuning [not in GPT-3, but mentioned as a future possibility]: Update weights of a pre-trained model by training on a task-specific supervised datasets; requires new large dataset for every task.
Few-shot learning: “Model is given a few demonstrations of the task at inference time as conditioning, but no weight updates are allowed”; majorly reduces need for task-specific data, but results can be much worse than SOTA fine-tuned models.
One-shot learning: Only one demonstration is allowed. “The reason to distinguish one-shot from few-shot and zero-shot is that it most closely matches the way in which some tasks are communicated to humans,” e.g. MTurk.
Zero-shot learning: No demonstrations are allowed. Model is only given natural language instruction for the task. Maximum convenience, robust, but “In some cases it may even be difficult for humans to understand the format of the task without prior examples, so this setting is in some cases ‘unfairly hard’.”
If you’re curious what GPT-3 is trained on:
It is surprisingly hard (200B+ parameters and not meeting 100% accuracy in most cases) to get GPT-3 to do basic math, which is not so much a critique of GPT as a reminder that current large language models are not tailored to every application. “GPT-3 displays reasonable proficiency at moderately complex arithmetic in few-shot, one-shot, and even zero-shot settings.”
I found this SAT analogy evaluation funny; few-shot GPT-3 with 100B+ parameters probably performs better on the SAT analogy section than I ever could.
This paper really starts to up the ante when it gets into the generated articles and human critical perception of machine- vs. human-written text. Intuitively, “People’s ability to identify whether news articles are model-generated (measured by the ratio of correct assignments to non-neutral assignments) decreases as model size increases,” approaching 50% (random chance) at a trillion parameters.
Importantly, the authors address data contamination: “Since our training dataset is sourced from the internet, it is possible that our model was trained on some of our benchmark test sets. Accurately detecting test contamination from internet-scale datasets is a new area of research without established best practices. While it is common practice to train large models without investigating contamination, given the increasing scale of pretraining datasets, we believe this issue is becoming increasingly important to attend to,” hence the cleaned [removed all potentially leaked examples, e.g. examples that have 13-gram overlap with anything in pretraining set] Common Crawl dataset. There is a bias and fairness analysis, but the authors make a measured statement that “internet-trained models have internet-scale biases” and present gender, race, and religion model examples as facts without getting into position statements on corporate responsibility.
GPT-3 is a 175 billion transformer-based language model that powers ChatGPT and catapulted LLMs and generative AI into the public eye. Whereas GPT-3 focused on text input and output, GPT-4 took it multimodal and introduced images (and GPT-4o with voice was OpenAI’s 2024 public claim to fame… and notoriety).
LLaMA: Open and Efficient Foundation Language Models (Meta, 2023)
ChatGPT’s explosive success without a doubt spurred executive-sanctioned research and development driving Google, Microsoft, and Meta to release Bard, Copilot, and Meta AI in rapid succession in 2023.
Meta released LLaMA (more easily typed Llama), a collection of foundation language models trained at 7-70B parameter scale, specifically calling out LLaMA-13B outperforming GPT-3 (while being 10x smaller). Meta’s training dataset is a bit more comprehensive across sources, including GitHub, arXiv, and StackExchange.
The authors also present work to quantify “bias, toxicity and misinformation”: RealToxicityPrompts
sends 100,000 prompts to the model, determines a toxicity score for each response via PerspectiveAPI; they propose WinoGender benchmarking and TruthfulQA.
Llama 3 was released April 2023 (8B-70B parameters) marketed as “a major leap over Llama 2”.
Mistral 7B (Mistral, 2023)
Mistral 7B outperforms the previous best 13B model (Llama 2, [26]) across all tested benchmarks, and surpasses the best 34B model (LLaMa 34B, [25]) in mathematics and code generation.
Mistral 7B leverages grouped-query attention (GQA) [1], and sliding window attention (SWA) [6, 3]. GQA significantly accelerates the inference speed, and also reduces the memory requirement during decoding, allowing for higher batch sizes hence higher throughput, a crucial factor for real-time applications. In addition, SWA is designed to handle longer sequences more effectively at a reduced computational cost, thereby alleviating a common limitation in LLMs. These attention mechanisms collectively contribute to the enhanced performance and efficiency of Mistral 7B.
By now it’s clear from the literature that training very large models requires very large computing power, and interweaving increasingly complex networks of computational nodes and memory machines is intrinsically exponential without optimization.
Mistral’s sliding window attention is rooted in fundamental software engineering principles, and the requisite rolling buffer cache a nod to scalable systems design. Just as OpenAI changed the game by identifying and addressing a limitation in large-scale tandem model and data availability, Mistral (and Meta, AWS, NVIDIA, and other hardware-oriented players) had the right idea to focus on computation- and complexity-saving architectural design while minimizing model performance compromises.
Gemini: A Family of Highly Capable Multimodal Models (Google, 2024)
Google’s competitor to GPT and Llama is Gemini (previously Bard), a powerful set of models in its own right and also the driver behind viral Google Search AI results.
Gemini Ultra is the first model to achieve human-expert performance on MMLU — a prominent benchmark testing knowledge and reasoning via a suite of exams — with a score above 90%.
Notably, Gemini is trained on TPUs (Tensor Processing Units), Google Cloud’s custom ASIC chip optimized for deep learning by addressing the von Neumann bottleneck of CPUs and GPUs via systolic array architecture (systolic arrays themselves share core principles with neural networks). [N.B. Humanizing and validating to see that even the Google Cloud Blog is not above directly linking off to Wikipedia pages]
Scaling Monosemanticity: Extracting Interpretable Features from Claude 3 Sonnet (Anthropic, 2024)
Anthropic’s firm stance as an safety-first company gives it massive brownie points in my book (I also like Anthropic’s website the most), but such claims do warrant due diligence into the technical workings. [I’ll try to stay unbiased by ignoring that Chris Olah and Shan Carter are listed authors for now, but that’s a mark of confidence. This is also far and away the most beautifully-written paper on this list.]
Eight months ago [paper publication date May 21, 2024], we demonstrated that sparse autoencoders could recover monosemantic features from a small one-layer transformer. At the time, a major concern was that this method might not scale feasibly to state-of-the-art transformers and, as a result, be unable to practically contribute to AI safety. Since then, scaling sparse autoencoders has been a major priority of the Anthropic interpretability team, and we're pleased to report extracting high-quality features from Claude 3 Sonnet, Anthropic's medium-sized production model.
Polysemanticity is a phenomenon that complicates the interpretation of neural networks (interpretability): “Individual neurons in neural networks often represent a mixture of unrelated features… when the input features are independent random variables that do not interact in the data-generating process, neurons that represent combinations of these input features can be confidently called polysemantic.”
Anthropic tackled this problem by driving towards monosemanticity with dictionary learning (formulated to counteract overcomplete models; also see disentanglement and superposition) and sparse autoencoders (an autoencoder is actually an unsupervised neural network employing backprop, designed to learn an identity function approximation— add a sparsity constraint to the hidden units and you have a controlled machine that learns about itself, on its own).
Famously, Anthropic uses an interpretable feature “The Golden Gate Bridge 34M/31164353: Descriptions of or references to the Golden Gate Bridge.”
The big leap that Anthropic makes with this paper is the longest, most extensive active treatment of safety and bias-mitigating features with a vision for how they can be applied and propagated at end consumer-scale. That’s huge.
If you’re interested in collaborating on future paper reviews or in-person paper reading sessions, please reach out on Twitter.
Bonus paper for wine geeks: FineWeb: decanting the web for the finest text data at scale (Hugging Face, 2024). Nice pun.